The Importance of Data Labeling in Machine Learning: A Comprehensive Guide
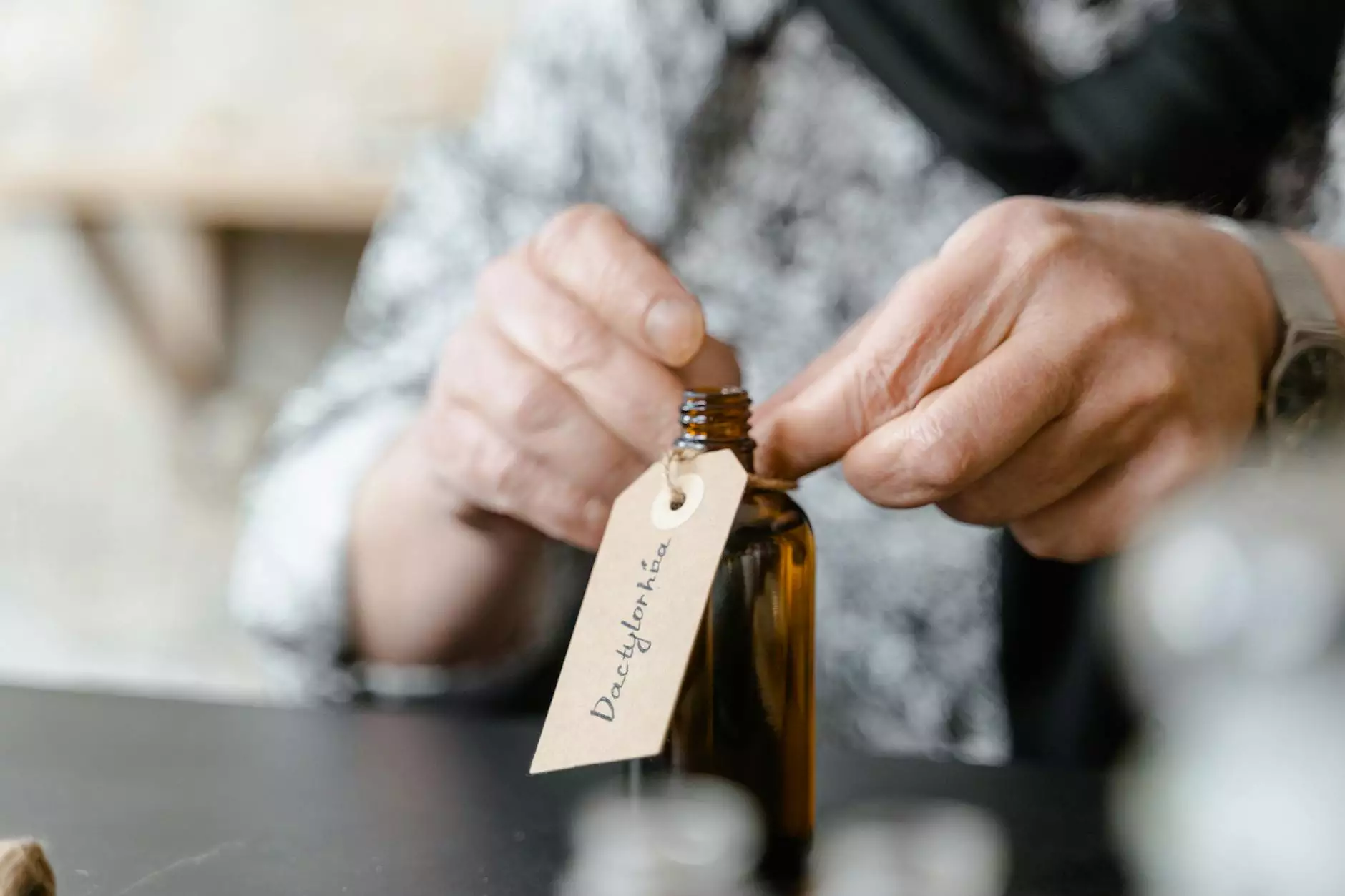
Introduction to Data Labeling and Machine Learning
In today's data-driven world, data labeling has emerged as a cornerstone in the development of effective machine learning models. As artificial intelligence (AI) technologies continue to evolve, the need for accurate and high-quality data annotations has never been more pressing. This article delves into the concept of data labeling machine learning, exploring its significance, methodologies, and the impact it has on various industries.
What is Data Labeling?
Data labeling refers to the process of annotating and tagging data to provide context for machine learning algorithms. This process involves identifying and categorizing elements within datasets, enabling machines to learn from and interpret the data accurately. The labeled data acts as a guiding framework for machine learning models, helping them to understand patterns and make decisions based on input data.
Types of Data That Require Labeling
The types of data that require labeling can be diverse and include:
- Text Data: This includes articles, reviews, and social media posts that need sentiment analysis or topic categorization.
- Image Data: Images require labeling for object recognition, segmentation, and classification tasks.
- Audio Data: Audio signals must be transcribed and annotated for speech recognition systems.
- Video Data: Videos need frame-by-frame annotation for tasks like action recognition and scene segmentation.
The Role of Data Labeling in Machine Learning
The role of data labeling in machine learning cannot be overstated. It provides the foundation for AI systems, enabling them to learn from historical data and make predictions based on new data inputs. There are several key benefits associated with effective data labeling:
1. Enhances Model Accuracy
Accurate data labeling directly correlates with improved model performance. When data is labeled correctly, machine learning algorithms can learn more effectively, resulting in higher accuracy rates. This is particularly important in high-stakes industries such as healthcare and finance, where erroneous predictions can lead to serious consequences.
2. Reduces Training Time
High-quality labeled datasets expedite the training process of machine learning models. Labeling data accurately from the outset minimizes the need for re-labeling or correcting errors later, allowing data scientists to focus on optimizing algorithms rather than fixing foundational issues.
3. Facilitates Better Decision Making
Well-labeled data leads to better insights. Organizations can utilize the patterns and information extracted from data to make informed decisions, enhancing strategic planning and operational efficiency. This is particularly crucial for businesses aiming to stay ahead of the competition.
Methodologies of Data Labeling
Various methodologies can be employed for data labeling. Each method has its advantages and disadvantages, depending on the context and requirements of the machine learning project. Here are a few common approaches:
1. Manual Data Labeling
Manual data labeling involves human annotators labeling data sets. While this method can yield high-quality data, it is often time-consuming and costly, particularly for large datasets. Moreover, the quality of the labels can vary significantly depending on the annotators' expertise and understanding of the task.
2. Automated Data Labeling
Automated data labeling utilizes algorithms to label data quickly and efficiently. This approach can significantly reduce costs and time associated with manual labeling. However, the accuracy of automated labeling still depends on the quality of the algorithm and may require human oversight to ensure precision.
3. Semi-Automated Data Labeling
This hybrid approach combines both manual and automated techniques. In semi-automated labeling, algorithms perform an initial pass on the data, and human annotators revise and correct the labels as necessary. This method balances speed and accuracy, making it a popular choice for many companies.
Choosing the Right Data Annotation Tool
Selecting the right data annotation tool is crucial for effective data labeling. The tool must align with your specific needs, including the type of data you are working with and the complexity of your project. Here are some key features to consider:
- Ease of Use: The interface should be user-friendly to facilitate quick training of annotators.
- Collaboration Features: Tools that support team collaboration can enhance productivity.
- Quality Assurance: Ensure the tool has mechanisms for quality checks to maintain high annotation standards.
- Integration Capabilities: Choose tools that can seamlessly integrate with your existing systems and workflows.
Data Annotation Platforms: Revolutionizing Data Labeling
Data annotation platforms provide comprehensive solutions for data labeling across various types of datasets. These platforms often facilitate both manual and automated labeling processes, allowing organizations to scale their data annotation efforts effectively. Key players in the market offer diverse features, catering to different industries and specific needs.
Key Features of Modern Data Annotation Platforms
- Scalability: They allow organizations to handle large volumes of data efficiently.
- Machine Learning Support: Many platforms integrate machine learning capabilities that learn from user inputs, enhancing future labeling processes.
- Clear Data Management: These platforms often include tools for managing and storing labeled datasets securely.
- Reporting and Analytics: Built-in reporting features help in tracking progress and quality control over the data labeling process.
Industry Applications of Data Labeling in Machine Learning
The applications of data labeling machine learning span numerous industries, demonstrating its versatility and importance. Here are some notable applications:
1. Healthcare
In healthcare, labeled data is essential for developing predictive analytics tools, diagnostic algorithms, and personalized medicine solutions. For instance, labeled medical imaging helps in training models that can detect diseases such as cancer, thereby improving patient outcomes and reducing costs.
2. Autonomous Vehicles
The automotive industry relies heavily on labeled datasets for training self-driving cars. Annotated data from various sensors enables these vehicles to recognize traffic signals, pedestrians, and road markings, ensuring safe and efficient navigation.
3. Retail
Retailers use data labeling for customer personalization and inventory management. By analyzing labeled transaction data, businesses can optimize their marketing strategies and enhance the customer experience by providing tailored recommendations.
4. Finance
The finance sector uses data labeling to detect fraudulent transactions and assess credit risk. Labeled historical transaction data trains algorithms to identify patterns indicative of fraud, helping organizations mitigate risks and improve regulatory compliance.
Challenges in Data Labeling
Despite its importance, data labeling comes with several challenges that organizations must navigate:
- Cost: Manual data labeling can be expensive, especially if high volumes of data are involved.
- Time-Consuming: The labeling process can be slow, impacting project timelines.
- Quality Control: Ensuring consistent quality across large datasets can be difficult, particularly with manual labeling.
- Skill Requirements: Specialized data labeling often requires expertise that may not be readily available.
Future of Data Labeling in Machine Learning
As the demand for accurate labeled data continues to grow, the future of data labeling machine learning looks promising. Innovations in AI and machine learning are driving the development of advanced data annotation techniques, including:
1. Enhanced Automation
Future data labeling systems are expected to incorporate more sophisticated automation, reducing the need for manual intervention and speeding up the labeling process while maintaining quality.
2. Crowdsourcing
Crowdsourcing data labeling can provide a scalable and cost-effective solution, leveraging a global pool of annotators to label data more efficiently.
3. Improved User Interfaces
As the industry matures, user interfaces for data annotation tools will become more intuitive, allowing non-technical users to engage in the data labeling process effectively.
Conclusion
In conclusion, data labeling machine learning is an essential process that drives the performance of machine learning models. As organizations recognize the value of high-quality labeled data, investing in robust data annotation tools and platforms becomes imperative. By overcoming challenges and leveraging advancements in technology, businesses can harness the full potential of their data, leading to enhanced decision-making, efficiency, and innovation in their respective fields. Embracing a strategic approach to data labeling can undoubtedly set organizations apart in the increasingly competitive landscape of AI and machine learning.